Publicly funded organisations seeking to maximise both insight and efficiency may want to experiment with generative AI. But many publicly funded organisations are concerned that instead of generating genuinely new insights, generative AI will draw on existing information and insights that reinforce the status quo rather than improving it. Even with the most noble intentions that aim for better outcomes, AI may fall short of that target.
Generative AI algorithms are trained on historical data and information generated from previous analyses. This means generative AI is inherently biased towards identifying known patterns and trends, which limits its ability to produce genuinely novel insights.
Many generative AI algorithms are also optimised to a specific goal, like reducing costs or increasing utilisation of valuable assets. Algorithms trained to focus on specific goals may not consider factors beyond the scope of the problem they are trying to solve, potentially overlooking other opportunities.
The quantity and quality of the data available to train generative AI algorithms can also limit effectiveness. Gaps in the data, poor data quality, or skewed data sets can lead to low-value analysis and insights.
If organisations come to rely too heavily on AI and the efficiencies it can generate, this can crowd out other sources of insight. Efficient AI-generated recycling of past insights may be a false economy in the longer term if organisations become less willing to experiment and take risks, leading to a stagnation of innovation.
Aggregating and extrapolating from historical information can also reinforce existing biases. For example, if data used to train an algorithm contains bias against a particular group or demographic, the algorithm may produce outputs that reinforce those biases. Despite giving the impression of impartiality, AI may perpetuate or even exacerbate inequity rather than addressing it. Publicly funded organisations charged with solving wicked problems need the power of innovation, not just aggregation.
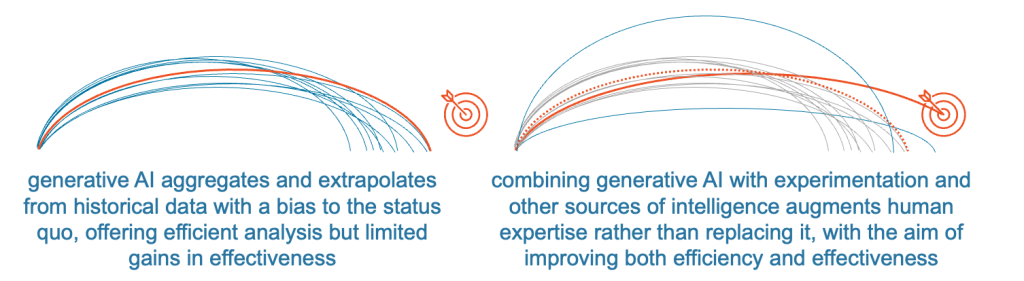
Generative AI should augment analysis, decisions, and experimentation, not replace them. Combining AI with other analytical techniques, like human expert analysis and multiple algorithms optimised for different goals, can be more effective and less risky. AI can process an impressive amount of data and information, but it is still a naive analyst, not an expert capable of contextualising and interpreting its findings.
Involving a diverse range of stakeholders in the design, deployment, and interpretation of generative AI algorithms can help to reduce inherent bias towards the status quo. The data used to train generative AI algorithms should also be as representative and diverse as possible, with both inputs and outputs scrutinised for biases and continuously improved.
Generative AI has the potential to efficiently generate insights, but it can also recycle and repackage well-known or simplistic insights in ways that reinforce the status quo and crowd out other sources of intelligence. It should be only one of many analytic tools used to augment human analysis and decision-making.
Ensuring that the data used to train algorithms is diverse and representative, continuously assessing and improving both inputs and outputs, and involving diverse stakeholders in design and deployment can help to avoid reinforcing existing biases.
By using generative AI to augment human expertise, organisations can more confidently use new analytic tools for experimentation that aims for both efficiency and effectiveness, and hits its target.